Good news: the era of manual data processing is over. Instead, we’re making way for the transformative power of automated data processing. In this article, we’ll dive into four game-changing practices that’ll revolutionize how you handle data in the financial services industry. Plus, we’ve got real-world examples to bring these practices to life and show you just how impactful they can be.
Gone are the days of painstakingly sifting through mountains of data, trying to extract meaningful insights. Now, using advanced technology, you can process and analyze crazy amounts of information. And, you can do it so fast, which leaves you more time to focus on the stuff that really matters. (Like making better decisions and driving growth.)
Research out of IDC predicts banking to be one of the top two industries spending the most on AI by 2024. And automated data processing is a key initiative among finance leaders.
We all know the pain of dealing with several data sources. Each comes stocked with its own quirks and idiosyncrasies. But automation can help you aggregate, cleanse, and harmonize even the most disparate datasets.
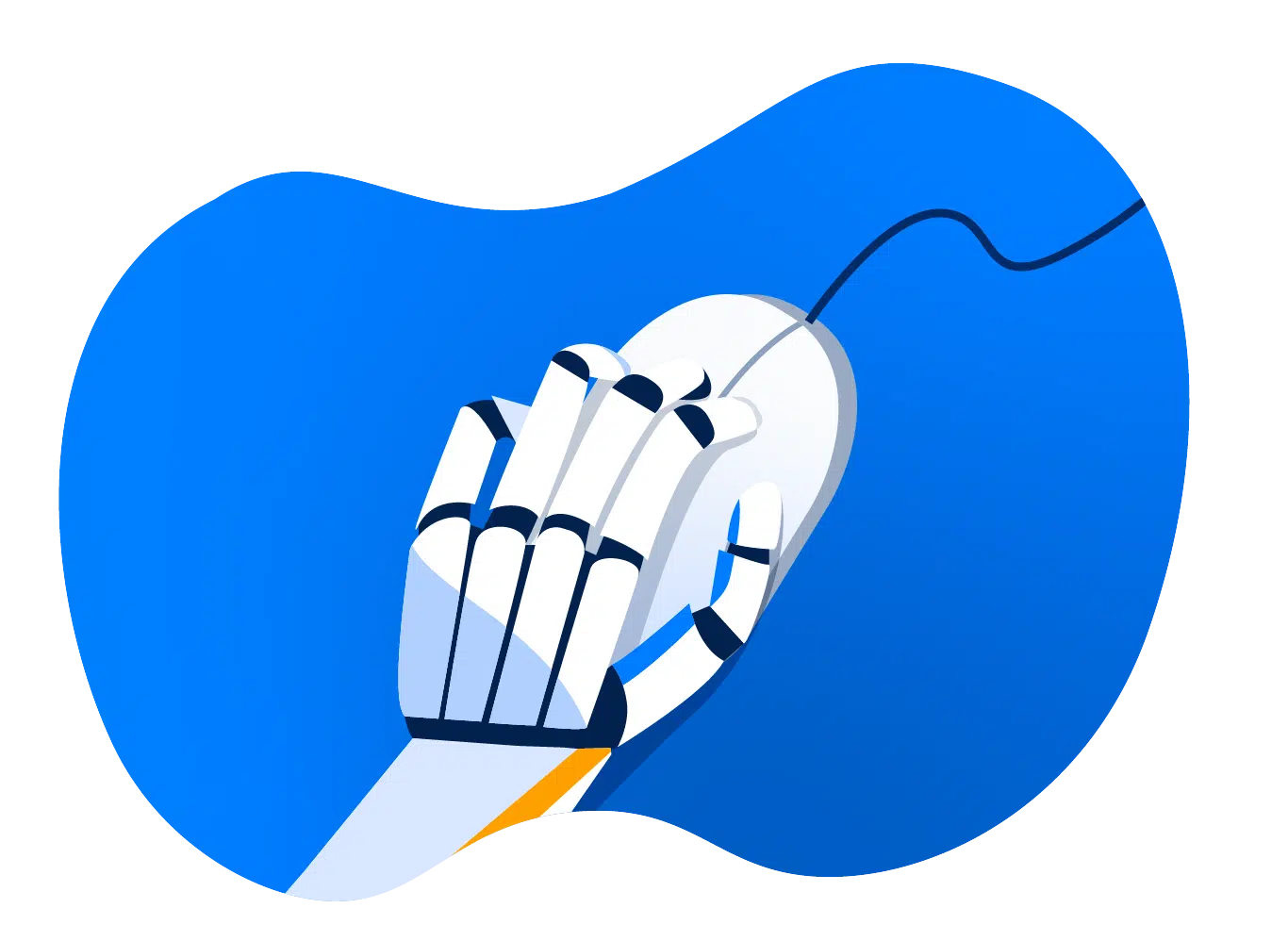
Automate Your Work
Capacity’s enterprise AI chatbot can help:
- Answer FAQs anytime, anywhere
- Find relevant documents within seconds
- Give surveys and collect feedback
Practice 1 – Data extraction and integration
Imagine a scenario where you effortlessly bring your data together from diverse sources. Whether it’s customer information, market data, or transactional records, you can collect it all in seconds. That’s the power of automated data extraction. By using cutting-edge technologies, you can grab data from all your systems and even external sources, too. No more wasting precious hours tediously copying and pasting, or worse, dealing with incompatible file formats. With automation, you’ll have the data you need at your fingertips, ready for analysis and decision-making.
Once you’ve gathered your data, it’s time for the real magic: integration. It’s no secret that reconciling your data formats and fixing your inconsistencies is, well, nothing short of a headache. But automated data processing helps by letting you connect and combine disparate datasets. You can intelligently map and transform your data, ensuring consistency across your fields. And you can then de-duplicate and find potential errors.
Real-world examples of data extraction and integration in action are a plenty. Take, for instance, a multinational bank that integrates data from its various branches worldwide. They merge customer records and transactional data in real-time with automation. This process gives them a holistic view of their customer base. And then it gives them a chance to increase their customer-specific offerings.
Or, consider a hedge fund that relies on automated data extraction to gather market data. They can look at stock exchanges, news feeds, and economic indicators with automation. By combining the data, they can generate real-time insights. And then they can ID emerging trends and make informed investment decisions faster than ever.
Practice 2 – Data Cleansing and Validation
Clean and accurate data is the backbone of reliable financial analysis. However, data inconsistencies, errors, and outliers are common challenges in large datasets. Manual data cleansing and validation processes are time-consuming and prone to human errors. Therefore, automating these processes is crucial for ensuring data quality and integrity.
Automated data cleansing and validation processes use advanced algorithms, pattern recognition techniques, and outlier detection methods to identify and rectify data errors and inconsistencies. By applying these automated techniques, financial institutions can streamline the process of identifying duplicate records, correcting formatting issues, validating data against predefined rules, and identifying and handling outliers.
For example, an investment firm can use automated data cleansing algorithms to identify and rectify discrepancies in their portfolio data. These algorithms can detect and resolve inconsistencies in portfolio valuation, missing or incorrect security identifiers, and inconsistent transaction data. By automating the data cleansing process, financial institutions can ensure that the analysis and reporting are based on reliable and error-free information, ultimately leading to more accurate investment decisions and risk management.
Practice 3 – Data Transformation and Aggregation
Raw data holds immense potential, but it needs to be transformed into meaningful insights for effective decision-making. However, transforming raw data into actionable insights is often complex and time-consuming, particularly when dealing with large volumes of data from disparate sources.
Automated data transformation techniques simplify this process by converting raw data into an easily interpretable and analyzable format. Data mapping, enrichment, and aggregation are common automated practices used in data transformation.
Data mapping involves defining the relationships between different data elements and standardizing them for consistency and compatibility. This automated process ensures that data from various sources are aligned, allowing for seamless aggregation and analysis. It enables financial institutions to consolidate data from multiple systems and databases, facilitating comprehensive reporting and analysis.
Data enrichment is another crucial aspect of data transformation. It involves augmenting raw data with additional information or attributes that enhance its value and usefulness for analysis. Financial institutions can automate data enrichment processes by integrating external data sources, such as market feeds, demographic data, or social media data, to gain deeper insights and enrich their analysis.
A financial institution analyzing customer demographics for targeted marketing campaigns can automate the data mapping to transform customer data from various sources into a consistent format. This automated data transformation allows for seamless aggregation and analysis of customer attributes, enabling the institution to gain valuable insights into its customer base and tailor their marketing strategies accordingly. According to Gartner, about 80% of finance leaders plan to implement RPA.
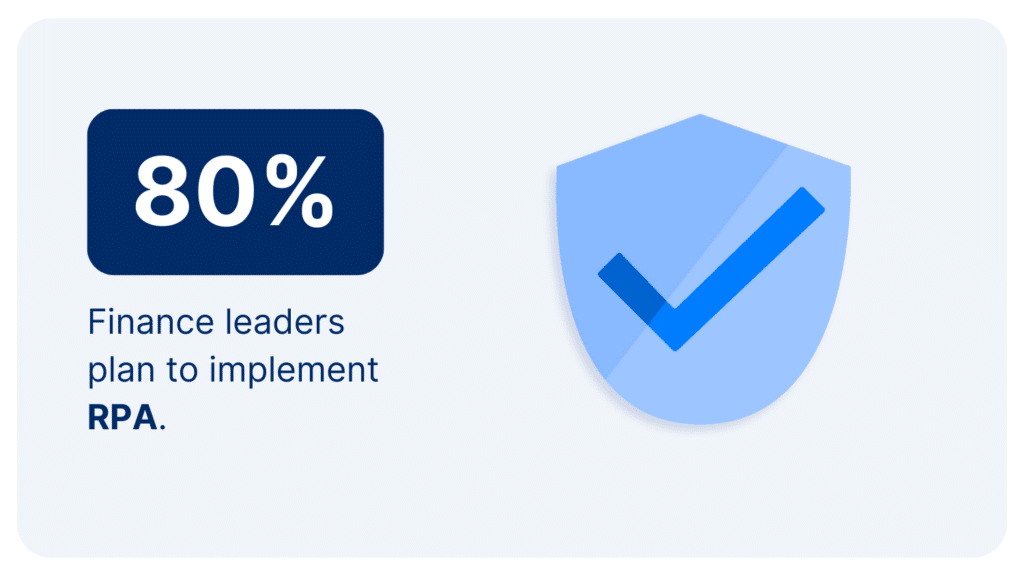
Practice 4 – Data Visualization and Reporting
Data visualization is vital in financial analytics, as it helps communicate complex insights clearly and concisely. Effective data visualization facilitates better understanding, enables decision-makers to identify trends and patterns quickly, and supports data-driven decision-making across various departments and functions.
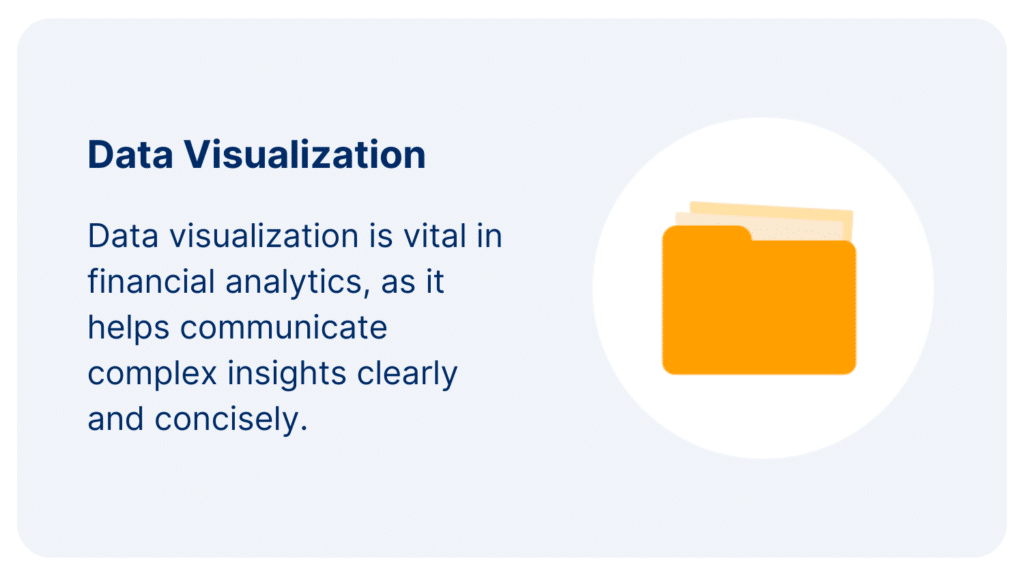
Automated data visualization tools and platforms have emerged as powerful solutions for creating interactive dashboards, reports, and visual data representations. These tools enable IT professionals in the financial services industry to transform raw data into compelling visualizations that convey information and insights effectively.
Financial institutions can tap automated data visualization tools to generate interactive dashboards that provide real-time insights into key performance indicators, financial metrics, risk factors, and customer behavior. These dashboards allow decision-makers to monitor business performance, identify emerging risks, and track the effectiveness of strategies and initiatives.
For instance, a risk management department can use automated data visualization tools to create visual representations of risk factors and trends. These visualizations provide a comprehensive overview of the institution’s risk exposure, allowing risk managers to make timely and well-informed decisions. By relying on interactive charts, graphs, and heat maps, risk managers can explore risk data at various levels of granularity and quickly identify areas that require attention or mitigation.
Automated data processing has revolutionized how financial institutions handle analytics, offering improved efficiency, accuracy, scalability, and decision-making capabilities. By implementing practices such as data extraction and integration, data cleansing and validation, data transformation and aggregation, and data visualization and reporting, IT professionals in the financial services industry can unlock the full potential of their data and gain unique insights. Embracing automated data processing empowers financial institutions to stay ahead in the competitive landscape, make informed decisions that drive business growth and success, and deliver exceptional value to their clients and stakeholders.
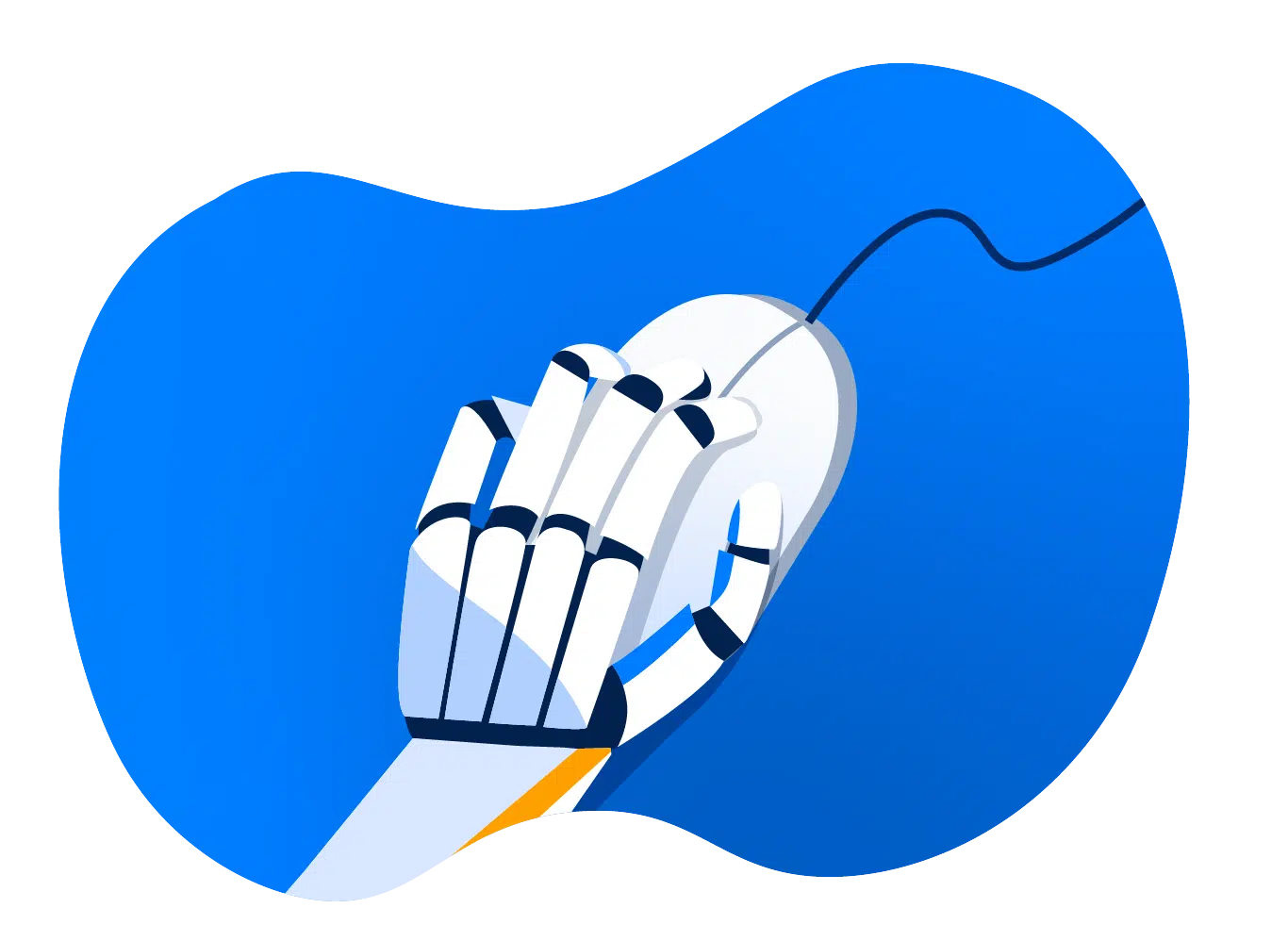
Automate Your Work
Capacity’s enterprise AI chatbot can help:
- Answer FAQs anytime, anywhere
- Find relevant documents within seconds
- Give surveys and collect feedback