Ethics
Ethics in AI
At Capacity, we are committed to ensuring that we responsibly bring AI into the workplace.

Why this matters
While AI is a powerful way to work smarter and reduce costs, it must be responsibly developed and implemented to ensure accuracy, efficacy, and safety.
Get the highlights
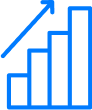
Higher ROI
Investing in a new tool shouldn’t be a risk. By prioritizing best data training practices, you’ll get superior results, fewer hallucinations and errors, and higher accuracy. This will ensure that you’re getting the most out of your investment.
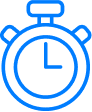
Faster Efficiency
When your tools work well, so do you. A well-trained and implemented AI tool will improve the way you work, not cause errors or delays.
Capacity’s Seven Guiding Principles

Principle 1
Look For Bias Everywhere
Data bias can affect every output of an AI system, and it can be found everywhere.
Principle 2
Assess All Possible Outcomes
To measure the magnitude of bias, we measure the probability of its occurrence against its overall impact, which helps prioritize where to start reducing it.
We use the formula: magnitude of bias = probability of occurrence x impact of bias.
For example, something could be a low bias severity but very likely to occur. Or an outcome could have a low probability of occurrence but a high impact.
We aim to weigh these two together when deciding where to spend time in reducing bias in AI systems.


Principle 3
Use Diverse Data Sets
An overabundance of one kind of data will erroneously skew results, so it’s better to have multiple diverse data sets.
A classic case involves training an image classifier to recognize animals (a common training task thanks to the availability of Imagenet). A particular algorithm kept scoring well on identifying closely related animals – like tigers vs lions. However, the algorithm kept failing on delineating between domesticated dogs and wolves. The researchers kept tweaking the algorithms and fine tuning the hyperparameters to no avail.
Eventually, a human team went in and started recognizing a pattern. If an image of a domesticated dog was in the mountains of Colorado, the algorithm incorrectly identified that dog as a wolf – not by the characteristics of the dog itself – but by the background snow! The research team quickly deduced that all of the wolf pictures in the training set were trained with snow in the background. Once they fixed the training set (by adding more diversity of wolves in other climates) the algorithm produced the intended results.

Principle 4
Reduce Bias with Self-Learning
User feedback helps the system learn and improve over time.
From early on, we recognized that a robust feedback mechanism is core to ensuring that Capacity is delivering great results. That’s why every response we provide is paired with thumbs up/down feedback. This feedback is fed directly into our neural networks to improve responses over time.
Principle 5
Diversify Trainers
People of various demographic backgrounds, as well as professional ones, will help the AI grow smarter and more comprehensive. For example, an accountant may have a completely different way of asking questions than a social media manager.


Principle 6
Collaborate to Reduce Bias
If one organization is experiencing AI-driven bias, it’s likely that others are too. It’s important to network with peers and learn how they are overcoming it. It’s also why we are part of Prepare.ai, a nonprofit (501c3) designed to champion connecting companies working on AI in the Midwest region.
Principle 7
Commit to Improving through Transparency
Contextualizing why an AI has provided an answer gives users confidence in the tool—they understand how the answer is relevant, rather than guessing why it was given.

Explore the Capacity platform
Trusted by 19,000+ organizations

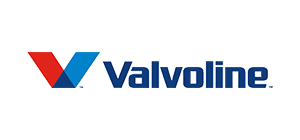
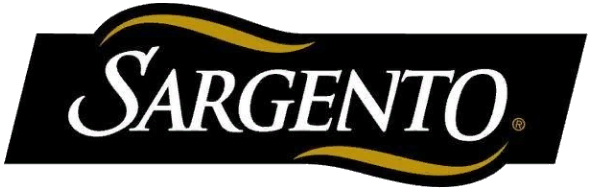
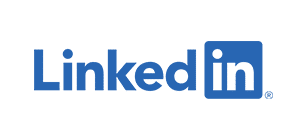








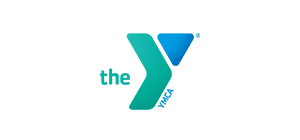


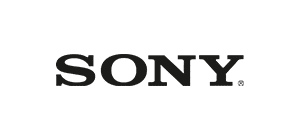


Ready to elevate every conversation?
Find out how Capacity can help your team do their best work.