As enterprises leave the 2010s and enter the 2020s, one thing that will remain the same: the need for a competitive advantage. Artificial intelligence has the potential to carry companies to the future of work, becoming more intelligent, more productive, and, potentially, more competitive.
However, even with the increased use of the term “artificial intelligence,” the technology itself is not currently as widespread throughout sophisticated industries as some might assume.
In 2015, Gartner found that only 10% of businesses had or were planning to use AI. In 2018, AI implementations had risen to 25%, and Gartner’s research found a 37% implementation rate among enterprises in 2019. In a span of around four years, we have seen a 270% increase in AI deployments. Businesses have been using AI technology to automate workflows and business processes, to drive data-driven decision making, and more.
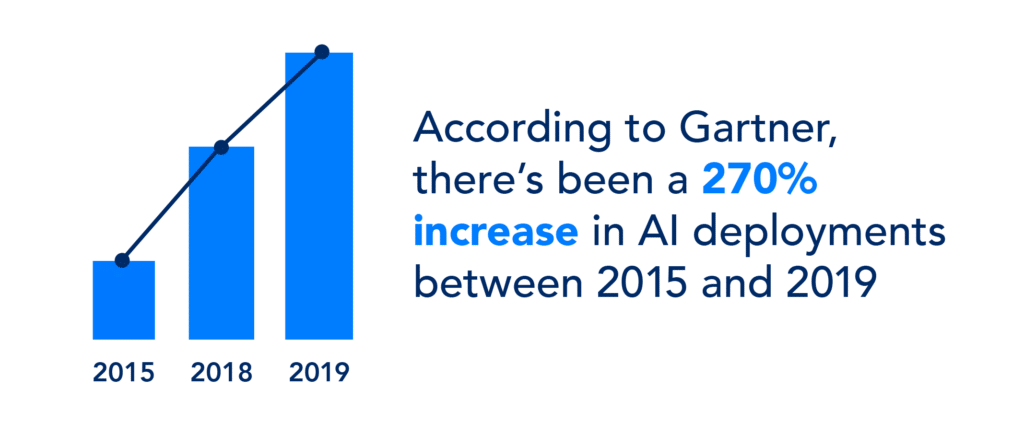
As with anything else that’s relatively new, organizations are still taking baby steps towards fully embracing AI. Although value proposition and participant buy-in represent decisive elements for any form of AI adoption, organizations will also need a developed and large data set so that they can test with AI technologies.
The participation numbers will vary from one study to another based on the report and the use cases. Regardless, AI is presently at the core of C-suite conversations. A study produced by the Economist Intelligence Unit found that nearly 75% of the 203 executives said that AI will be widely deployed within their companies during the next three years.
The notion of physical devices emerging as sentient beings has been pondered for decades. The ancient Greeks had their own robot speculations, and pioneers from China and Egypt designed automatons.
The origins of contemporary AI can be attributed to the efforts on the part of classical philosophers who started by characterizing human thought as a conceptual framework. Nonetheless, the theory of AI was not officially recognized until 1956, when the word “artificial intelligence” was proposed at a Dartmouth College conference.
In addition, Alan Turing was considered the pioneer of AI throughout his much-heralded research during the 1950s. In fact, he created the Turing Test to determine whether machines could form thoughts. His test required someone to write questions to both a machine and a human. If the interviewer couldn’t tell the difference between the answers from the machine or the human, then the computer would have passed the test.
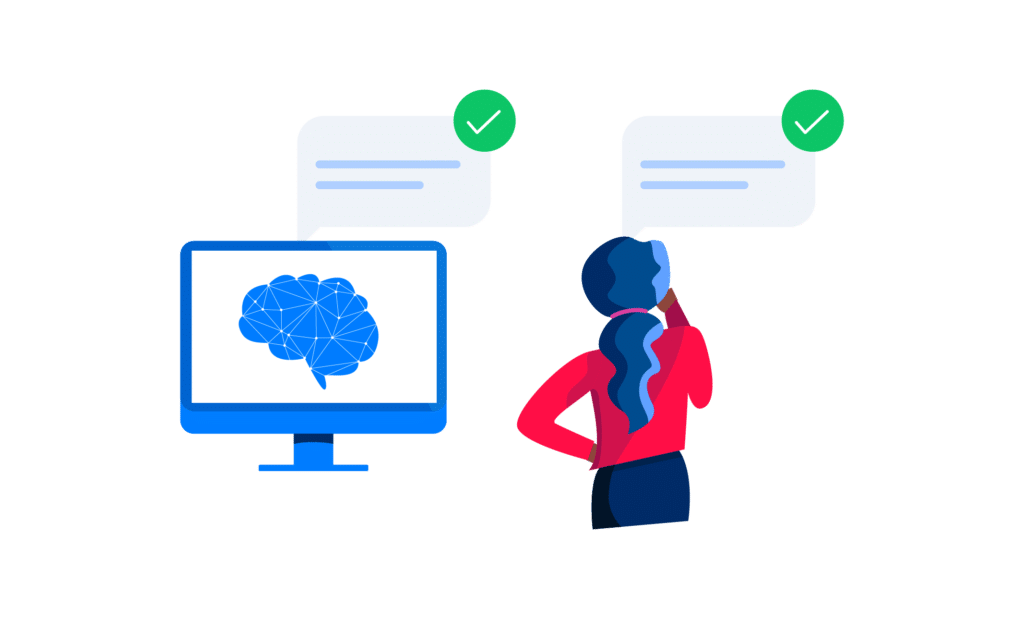
However, AI certainly has certainly had its growing pains. The years between 1974 to 1980 were labeled the “AI Winter,” after government funding and interest dropped due to several negative reports. Fortunately, the field was revived in the late 1980s with funding pouring in from the Japanese and the British government.
By the time we had reached the 1990s and 2000s, many historic AI milestones had been met. In 1997, IBM’s Deep Blue, a chess-playing machine, beat world champion and grandmaster Gary Kasparov. This high profile game would be the first time a defending World Chess Champion lost to a machine, and it served as a significant leap forward in terms of AI-driven decision making.
Further, a speech recognition program developed by Dragon Systems was implemented on Windows in the same year. The program demonstrated another major AI leap, but this time towards the challenge of decoding spoken languages.
Soon, it appeared that machines could be taught to handle anything. Even human emotion was replicated as shown in the example of Kismet, Cynthia Breazel’s robot creation that could identify and emulate emotions.
We now live in the “big data” period, an era in which we are able to accumulate vast quantities of data which are then too overwhelming for a human to manage. In many industries like technology, marketing, banking, and streaming services, the implementation of artificial intelligence in this respect has now been quite constructive.