AI is the Roy Kent of today’s business world. (S/O to my fellow Ted Lasso fans.) It’s here, it’s there. It’s every-(edited)-ing-where.
But the way the world is jumping headfirst into automation, it’s important to remember: AI is a tool. That’s it. Full stop.
Sure, it could be the Adidas Al Hilm used in the 2022 FIFA World Cup final. But at the end of the day, it’s a tool. And it’s only as useful and powerful and accurate as the person using it.
In 2019, some 85% of AI projects failed, with 96% of organizations reporting that they had problems with data quality, labeling, and building model confidence, according to TechRepublic. That’s why it’s so important for your IT department to make sure your AI project manager is set up with the right knowledge and the right skills to bury the shot into the back of the net, so to speak. The PM has to be set up for success.
To help you get started, here’s a rundown of everything an effective AI project manager should know and do.
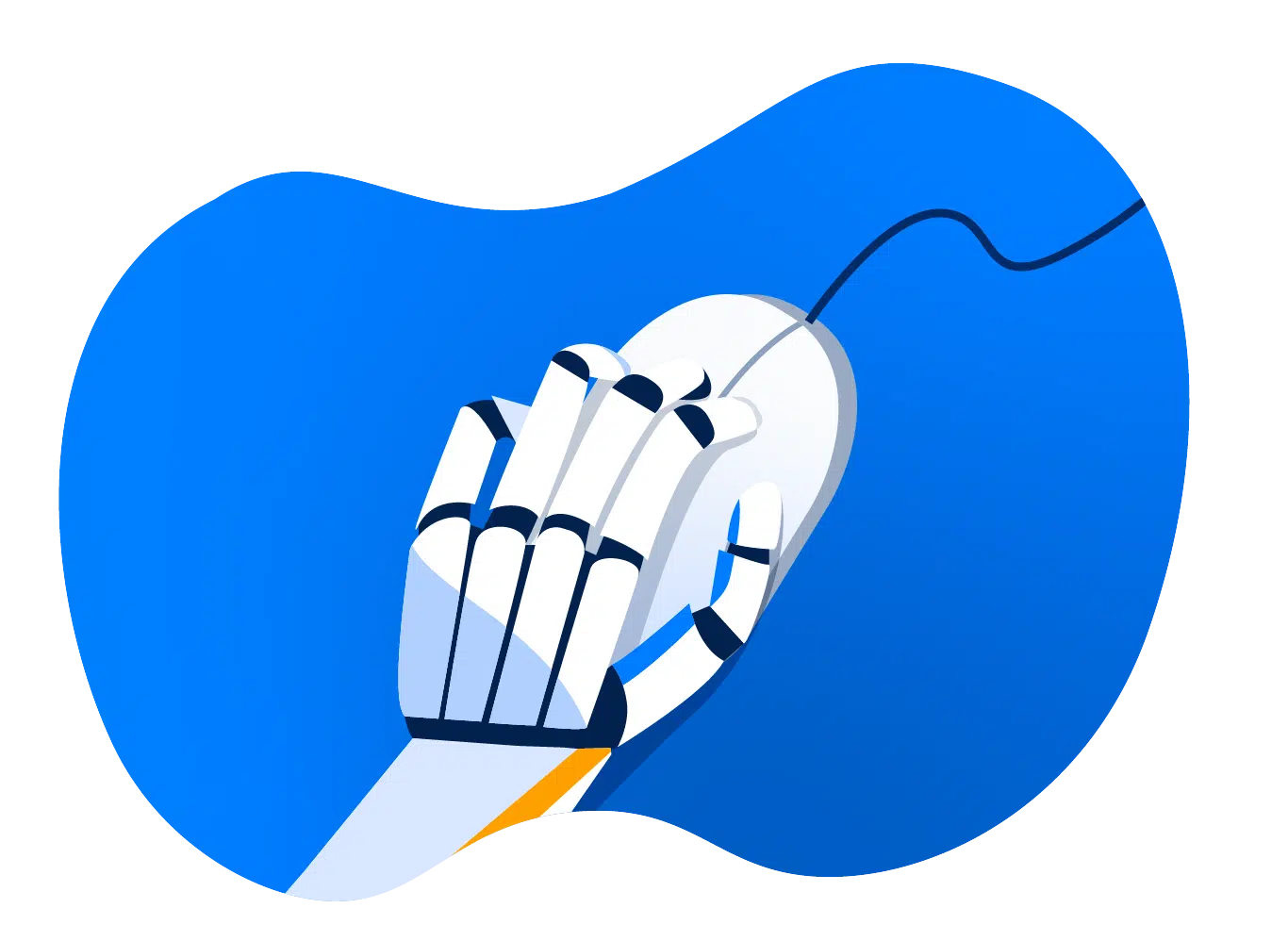
Automate Your Work
Capacity’s enterprise AI chatbot can help:
- Answer FAQs anytime, anywhere
- Find relevant documents within seconds
- Give surveys and collect feedback
Understand the Technology (and when to use it)
First and foremost, an AI project manager has to understand the tech behind AI. You should know the difference between a chatbot and an AI-powered customer service agent and when to use each.
You should also understand more complex concepts like natural language processing, machine learning, and deep learning. Knowing what each can do will help you at game time to decide whether they’re the right fit for your project.
Here are a few specific tools can consider adding to your automation playbook:
– Chatbots. You can use these to automate sales and customer service conversations.
– AI-powered customer service agents. Your service team can add these to provide personalized customer service experiences (without adding headcount).
– AI-powered helpdesks. Used these to deflect tickets, answer repetitive questions, and automate service.
– Data Visualization Platforms. These let you analyze and understand large amounts of data to ID actionable insights.
– Robotic Process Automation. You can use these to automate your business operations and workflows, mimicking human actions without actually passing to a human.
– Natural Language Processing. You can use NLP to let your AI systems understand human language and respond accordingly.
– Machine Learning. This is used to create models that can predict outcomes and make decisions autonomously.
– Deep Learning. Use this to create complex models that can analyze unstructured data and extract meaningful insights.
Knowing the tools you can use is quite literally just the first step. Next, you’ll want to define and document your project scope and goals.
Defining your project scope and goals
What is it that you’re actually trying to do with AI? How will you (and your CEO) define success? As an AI project manager, you need to establish a clear understanding of what you want to achieve and then how you plan to achieve it. The scope of your project should be well-defined. And it should also be well-documented to ensure everyone is on the same page.
Here are five things to think about as you define the scope and goals of your AI project.
1. What are your business needs?
What problem are you trying to solve with AI? How can automation help you achieve your goals? You should clearly establish exactly what your company’s goals, pain points, and use cases are for AI before you add it to any process. This will help you set realistic and achievable goals for your project.
2. What are the data requirements?
To build an effective AI system, you need data. So, you’ll need to define the data you need to collect, where you’ll get it from, and how you’ll store and manage it. And, you’ll need to ensure the data you’re collecting is relevant and sufficient to train your AI models. Data quality is critical, so you need to ensure that the data is accurate, complete, and free from bias.
3. Understand your technical requirements.
Define your hardware and software requirements. Then, outline the infrastructure and tools you’ll need to achieve your goals. You’ll also need to think about how you’ll develop, test and deploy your AI systems, as well as the technical requirements for said testing.
4. Know your risks.
Every project has risks, and AI projects are no exception. Identify and assess the risks associated with your project and create strategies to mitigate them. Specifically, consider the risks associated with data privacy, security, and ethical considerations.
5. Define your success metrics.
How will you know your AI project is working? What criteria are you setting up to say, “Yup, that’s right on track,” or “Whoa, I have some optimizing to do?” Set up clear and measurable goals and KPIs to track your progress throughout implementation and beyond. Also, consider whether your success criteria align with your company’s business goals. You’ll get more executive buy-in that way.
So what happens if you don’t hit your success metrics? What will you change? As you build out your roadmap and strategy, have a plan in place to constantly improve.
How to create a plan for optimizing your project
Start by collecting feedback from your stakeholders and end-users. Is automation making life easier? Does it help your company save time or money? Does it align with your organizational goals? As you collect feedback, use it to refine the scope of your project and adjust accordingly.
You should also regularly monitor your AI system’s performance. Analyze data points like accuracy, runtime, throughput, latency, and more to ensure that your system is running as expected. And if it’s not performing, what adjustments can you make? Make sure you’re running experiments and testing new versions of your AI model to ensure it’s working at its best.
Finally, take advantage of AI tools whenever possible. Automation can help reduce the amount of manual labor needed to manage your AI system, and then you can focus your energy on strategy and optimization.
AI project management is a big job; it’s not for the faint of heart. To be successful as an AI project manager, you need to have a solid strategy in place.
Get started with AI today (for free) with Capacity!