Depending on your business needs, AI can be used in a variety of practical ways. For instance, you can use AI for many things from pulling social data to improving logistics in terms of tracking and handling inventory or increasing engagement through your customer relationship management (CRM) platform.
But, as with any new technology, there will be challenges.
Managing transcription errors with speech recognition.
Let’s face it, speech recognition has come a long way, but there are still a few kinks to work out. Some of the most common errors include:
- Verbosity: Too many words added.
- Pronunciation: Incorrect pronunciation.
- Deletions: Removing words that were spoken.
- Issues with homonyms.
- Words are included that appear to make no sense.
- Misspellings.
- Uncategorized errors such as suffix errors.
Facial recognition misidentifies people outside of strictly controlled environments.
When the American Civil Liberties (ACLU) ran a test using the AWS Rekognition service, they found that 28 members of the U.S. Congress were incorrectly aligned with mug shots easily retrieved from public arrest photos. These types of shortcomings with facial recognition systems are disconcerting.
It’s important to recognize these types of miscalculations with facial recognition before more of these types of systems get deployed throughout various agencies. While they can certainly augment security and law enforcement, there are still some issues around misidentification.
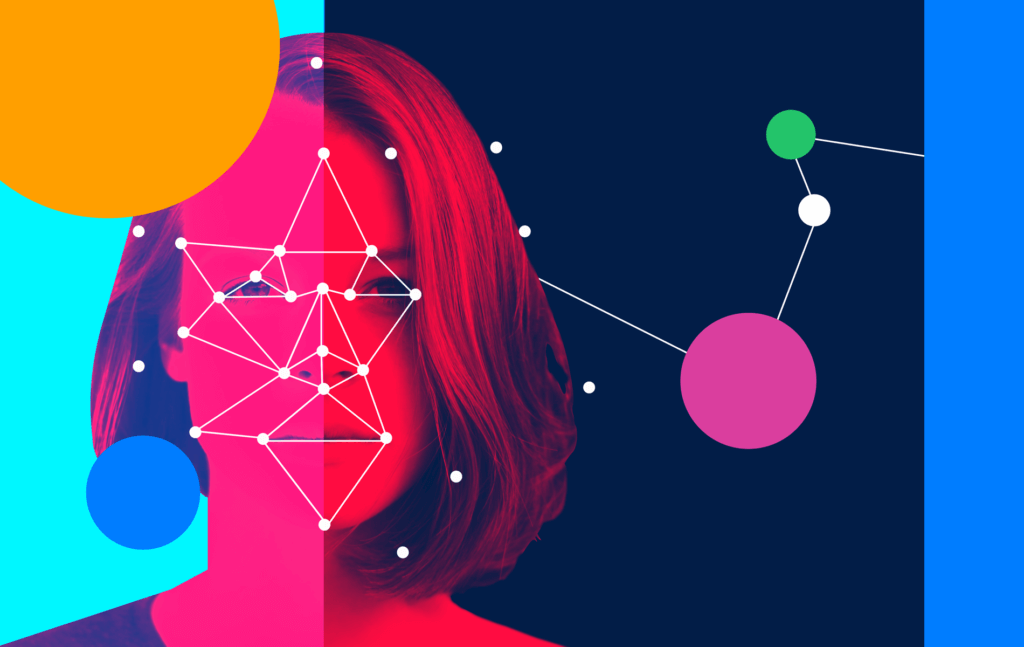
Full automation isn’t feasible for now.
A wide range of companies can agree that there are many business benefits that come with implementing AI. And, yet, there are still many organizations that can’t seem to decipher the technology, continuing to fear its implications. Naturally, every company is unique and will have a different AI adoption experience.
Data quality.
For starters, AI needs to identify patterns. The only way to accomplish this feat is by way of huge training data sets. Then, AI can learn similarly to humans. Since AI has the power of speed, it can learn rather quickly. But it feeds off data, and that data must be of acceptable quality to get good results.
Non-technical employees may not understand the purpose of AI.
Right now, AI deployment requires an understanding that may not be fully realized yet with every employee. In fact, AI needs to be comprehended from the viewpoints of both its possibilities and limitations. Still, the myths about AI abound such as needing to hire an in-house data science team to manage the AI.
Myths that AI is Skynet and will end humanity as we know it still abound. And, with a lack of AI knowledge comes a lack of deployment. On the other end of the spectrum, some people set their expectations too high and they believe AI will instantly transform their operations into an episode of Star Trek.
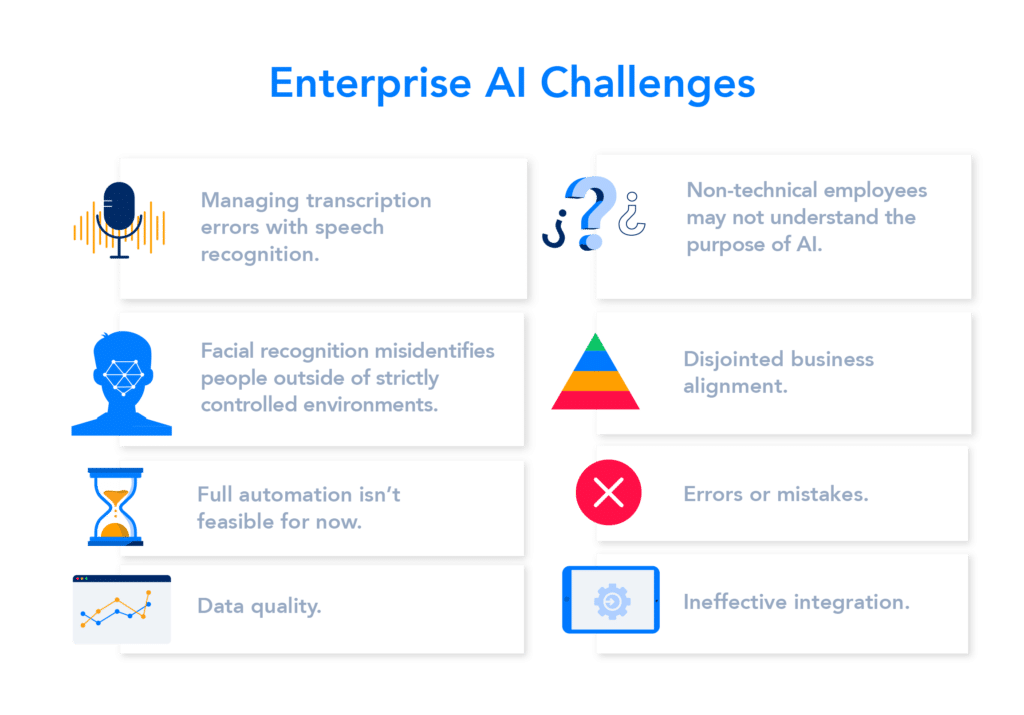
Disjointed business alignment.
A successful AI implementation requires a clear strategy, strategic methodologies, goal setting, KPIs, and ROI tracking. You can’t put the pedal to the metal and expect the AI cards to fall where they may. Don’t jump in without a plan. Further, identify business use cases.
Errors or mistakes.
Since AI learns so quickly, it can be difficult to determine where things went haywire. If AI makes a mistake, can you identify the cause or the root of the issue? Yes, it is intelligence, but it can also make mistakes. For example, human prejudices can penetrate its algorithms. The result is you end up with AI biases. Not to mention, with biases will come inaccuracies around predictions. In the AI world, this would be termed as “bad reasoning.”
Ineffective integration.
When you decide on AI integration, you have to understand that this is a lot more than simply installing a new plugin. Things to think about include what types of data sets you will feed into the AI system, labeling, data infrastructure needs, and data storage. After that, there is training and testing involved. For example, you have to test the data to ensure better accuracy. And, other tests need to be designed to determine whether the AI solution is working as intended or needs to be tweaked. And, if it needs to be adjusted, how will you do that?
How can you better manage the challenges of AI adoption?
To address any potential AI challenges, it helps to start with a strategy. You must also remember that you don’t have to resolve all the issues by yourself. Understand the process and recognize what types of possible issues may surface. It necessitates a step-by-step approach. A bug-free implementation may not be possible at this time. While there isn’t such a thing as perfection (yet), being prepared and proactive will give you the advantage.